ABGP - I Workshop AI applied to The Petroleum Industry
The use of deep neural networks in geoscience is already a reality and its applications range from single 1D well log prediction to more complex 3D earth model, including horizon and faults interpretation, facies and reservoir rock properties, etc. If the CNN architecture and fine-tuning of its hyper parameters play a key role in the success of the algorithm, it is of prime importance to be able to train the CNN on a significant amount of data with a wide range of possible scenarios.
Unfortunately, the Oil & Gas domain provides very few true samples of the 3D earth model, with a limited number of wells sparsely distributed laterally. Even at production-scale, an offshore O&G field often count only tens of wells across a large area. One way to mitigate this problem is to feed the Convolutional Neural Network with realistic synthetic data, that contains all the diversity of input/output pairs the user wants to predict. A key benefit of the synthetics is the huge amount of data that is possible to create in a very reasonable amount of time, and the possibility for geoscientists to control the validity of these different scenarios. The proposal of this work is to test the capacity of a Convolutional Neural Network to predict lithology directly from a seismic gather when trained with synthetics created from pseudo-wells.
Unfortunately, the Oil & Gas domain provides very few true samples of the 3D earth model, with a limited number of wells sparsely distributed laterally. Even at production-scale, an offshore O&G field often count only tens of wells across a large area. One way to mitigate this problem is to feed the Convolutional Neural Network with realistic synthetic data, that contains all the diversity of input/output pairs the user wants to predict. A key benefit of the synthetics is the huge amount of data that is possible to create in a very reasonable amount of time, and the possibility for geoscientists to control the validity of these different scenarios. The proposal of this work is to test the capacity of a Convolutional Neural Network to predict lithology directly from a seismic gather when trained with synthetics created from pseudo-wells.
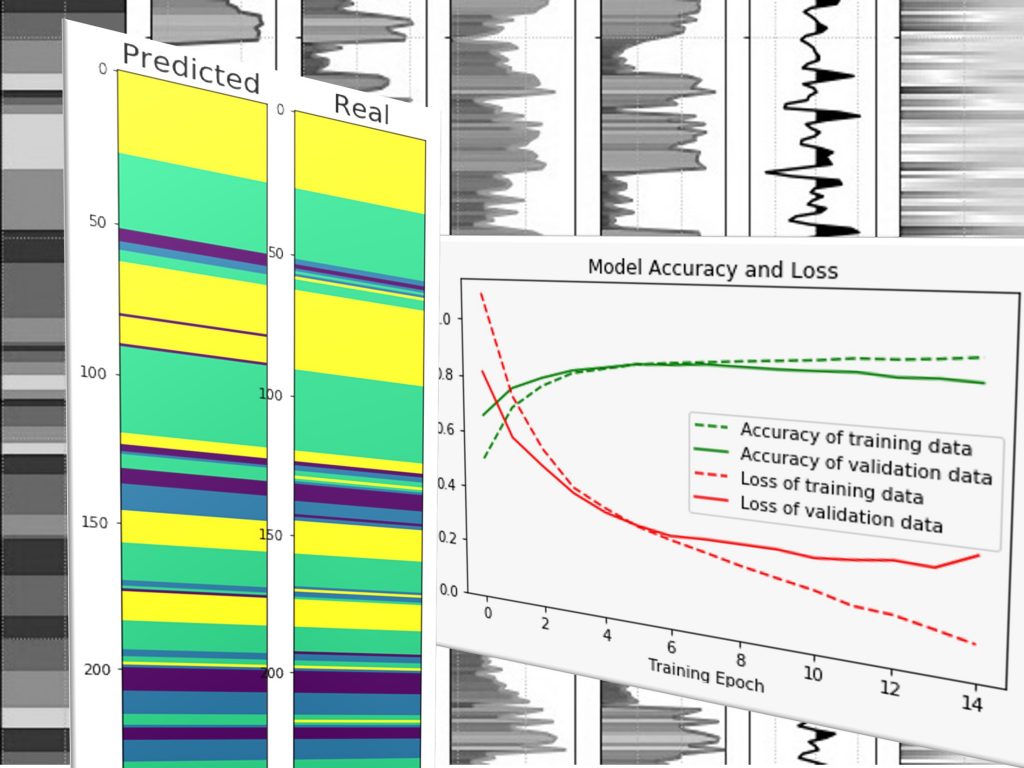