EAGE Conference on Seismic Interpretation using AI Methods
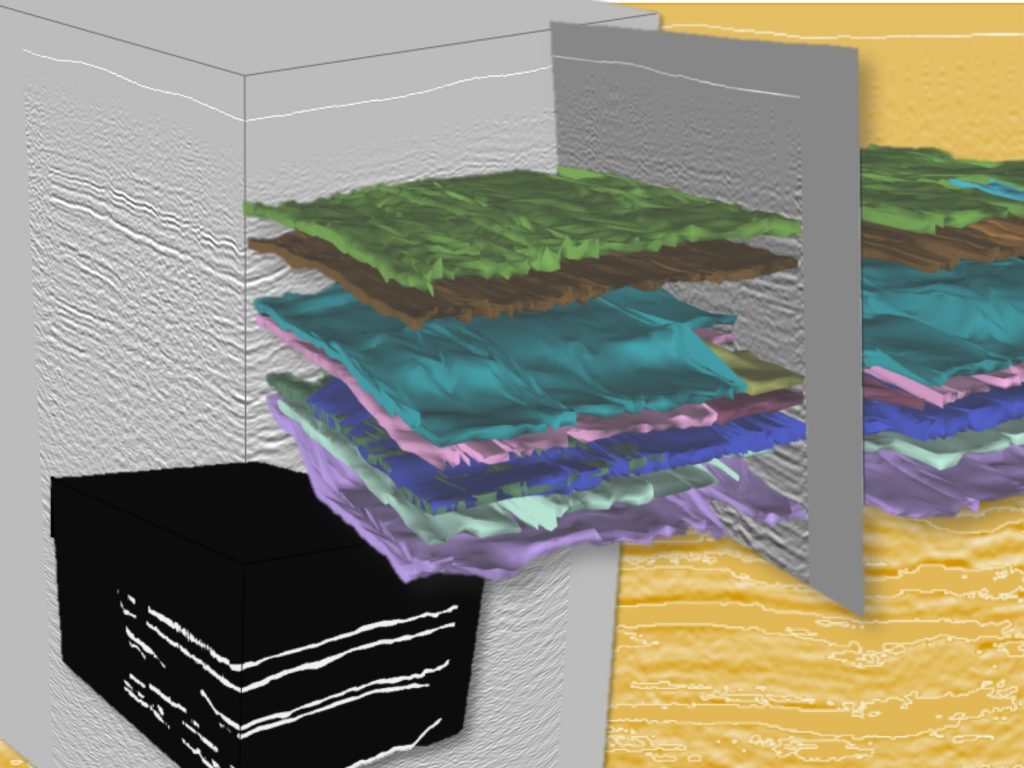
In a first step, an RGT model was built from the original 3d seismic data. This volume is obtained semi-automatically during an initial seismic interpretation phase by auto-tracking all possible horizons in the seismic volume, refining, and ordering their relationships. The resulting 3D cube represents relative geological ages, ranging from 0 (the shallower level) to 1 (the deeper level).
In parallel, a tedious manual interpretation of each diabase sill was performed (Figure 1) on the entire cube and produced a property volume of categorical data distinguishing igneous intrusions (value = 1) from the rest of the basin infilling (value = 0). The result of this work serves as a Mask to train the CNN to classify each seismic voxel as igneous or non-igneous rock.
A unique regional 2D line was then randomly selected along the cube, on which we extracted the three pre-processed data (amplitude, RGT, Mask) to serve as a training dataset for the Convolutional Neural Network algorithm.
The CNN architecture we used was derived from Waldeland et al. (2018) and comprises a classical succession of five 2d-convolutional layers and max-pooling, applied on 64×64 squared images. A binary cross entropy loss function with Adam optimizer was used to obtain the final classification result. Two independent CNNs were trained using the same hyper-parameters and training dataset:
1) the first one with only the amplitude as input (single channel)
2) the second one with both the amplitude and the RGT as input (two channels)
The CNN prediction was computed on the entire seismic cube along each Inline (2D ½) with both methodologies. Note that we limited on purpose the quantity of Training data to reproduce a practical situation where we often lack input training information.
Visually it is possible to see that the results of the single channel had poor lateral continuity and were significantly affected by the signal/noise ratio of the original seismic data. On the other hand, the multi-channel approach dramatically reduces the number of false positive and produces a more coherent geological output.
As conclusions, integrating the RGT model as a complementary input for the CNN classification model could substantially improve the sills prediction in the Parnaíba basin, where intrusions are sub-parallel to the deposition. This advantage can be beneficial for practical application when training dataset is scarce and needs to be optimized as much as possible.
Finally, the resulting sill prediction could serve as input to still improve the original RGT model in an iterative process of Global Seismic interpretation workflow.